Artificial intelligence (AI) was introduced in the 1950s when researchers first began exploring the idea of building intelligent machines that could mimic human problem-solving and decision-making. In these early days, AI was primarily focused on rule-based systems, which relied on predetermined rules to make decisions.
Over the past 70 years and particularly in the last two decades, AI has made significant strides. This progress has been supported by advances in cloud computing, computing speed, power, and memory. Additionally, it has been facilitated by the rise of big data, and the evolution of deep learning.
The emergence of these developments has opened up new applications for AI, enabling it to tackle increasingly complex problems with greater accuracy and efficiency.
As a result, AI has reached a pivotal point. Today, organizations across all industries are turning to AI-based technologies to improve their operations and revolutionize the way work gets done. According to Mckinsey’s 2022 AI survey, AI adoption has more than doubled since 2017, and the average number of AI capabilities that organizations use has increased by 50% since 2018.
AI Across Industries
AI is making significant progress commercially, as companies across all sectors are applying AI to improve their technology. Major organizations are using the technology across their suite of products. Let’s take a look at some examples:
1. Tesla: Tesla applies AI to its Autopilot by using deep neural networks to analyze sensor data and make accurate decisions about how the vehicle should respond. Tesla’s networks learn from a wide range of complicated scenarios sourced from their global fleet of vehicles. Their full build of Autopilot neural networks involves 48 networks that take 70,000 GPU hours to train.
2. Google: Google used AI to create its own “universal language” for Google Translate. Google has implemented their machine learning system, Google Neural Machine Translation (GNMT), to analyze languages by looking at full sentences instead of individual words.
This was the first time that machine based translation has successfully translated sentences using knowledge gained from training to translate other languages. As a result, multilingual systems are involved in the translation of 10 of the 16 newest language pairs.
3. Amazon: Amazon has leveraged AI to enhance its ability to provide ML-powered personalized recommendations to customers. Amazon uses an algorithm that they call “item-based collaborative filtering”, which analyzes customer data and connects purchase history with browsing data. This highlights the power of deep learning to go beyond object recognition and language processing to search and recommendations.
AI and Industrial Autonomous Vehicles
Artificial Intelligence has made significant contributions to the development of industrial autonomous vehicles. As a result, robotics and automation will account for more than 25% of capital spending over the next five years for industrial companies, according to the 2o22 McKinsey Global Industrial Robotics survey.
At Cyngn, we have leveraged advancements in AI to enhance our Enterprise Autonomy Suite (EAS). We use learning-based approaches for computer vision to analyze, interpret, and understand sensor data, including deep learning and machine learning (ML) models that can learn and improve from experience.
In particular, AI brings advantages to the perception modules of autonomous vehicles — object detection and classification. By utilizing AI, Cyngn is able to achieve:
1. Safe and efficient driving in dynamic environments
2. Completion of unique tasks
3. Semantic segmentation for accurate localization
4. Cheaper and faster deployment bring-up
-
How does AI help contribute to safe and efficient driving in dynamic environments?
AI-powered multi-modal object detection with granular classification is instrumental in helping our industrial autonomous vehicles safely and efficiently navigate dynamic environments and detect dynamic objects.
Here’s how it works:
First, the vehicles gather incoming raw data from lidar and cameras and use that data to identify objects in their environment. AI-based computer vision enables the vehicles to go beyond simple detection and provide detailed object classes for the objects identified. These classes yield insights that can inform autonomous driving decisions such as how to interact with a particular type of vehicle or the relevant rules associated with a human working near the vehicle.
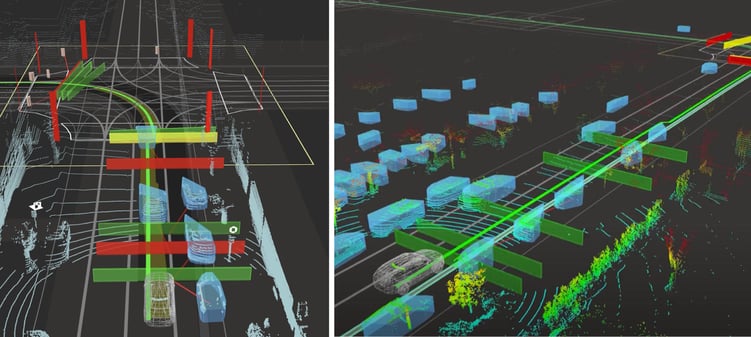
These images show how a warehouse robot processes the information on its route based on the semantic map and route plan. They also display the vehicle’s ability to detect stationary and moving objects in its path to make decisions.
“Multi-modal object classification unlocks the ability to prescribe driving behaviors that vary based on the type of objects present in the immediate environment,” says Kyle Stanhouse, Cyngn’s Director of Engineering and Autonomy. As a result, our warehouse robots can behave differently, depending on whether it is interacting with another vehicle, a pallet of goods, or workers.
Additionally, AI-driven object classification significantly improves the vehicle's ability to track and predict the behavior of objects over time, enabling more sophisticated decision-making in dynamic environments. This is especially important for defensive driving and creating autonomous vehicles that prevent potential driving risks as opposed to simply reacting to avoid collisions.
-
How does AI help autonomous vehicles complete unique tasks?
By enhancing perception, AI not only improves a vehicle’s ability to autonomously drive but also allows Cyngn to introduce unique automation tasks to our DriveMod-enabled vehicles. A unique task is defined as a specialized job outside of autonomous navigation.
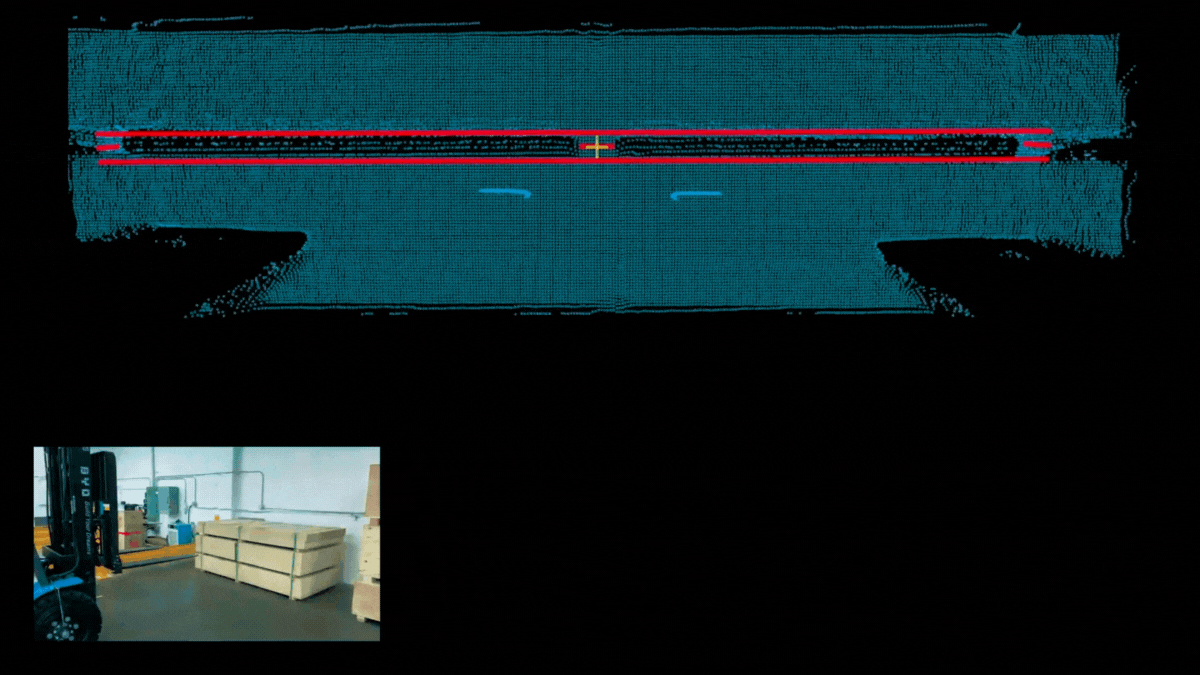
In this video, the autonomous forklift visualizes and accurately lines itself up with the pallet stack using on-vehicle sensors.
One example is using an autonomous forklift for pallet picking and placing. Leveraging AI, an autonomous forklift can accurately detect several crucial attributes about pallet stacks and the individual pallets within stacks based on real-time data from the on-vehicle sensors.
These AI insights can then be combined with other logic to determine important downstream AV functions like at what angle the forklift should approach a pallet stack, how many pallets are in the stack, and where exactly to position the forks to center the load. This results in a safe and reliable automated process.
-
How does AI-powered semantic segmentation make localization better?
AI-powered semantic segmentation is a powerful technique in computer vision to classify underlying elements (e.g. pixels in a camera image, points in a lidar point cloud) and segment various objects and regions within an image or video.
For instance, we can use semantic segmentation to identify the source of a particular feature associated with an object, allowing our mapping and localization system to isolate specific landmarks or salient features such as shelving units or walls.
By accurately identifying and localizing these features, AVs are able to navigate through semi-unstructured and dynamic environments with greater precision and safety.
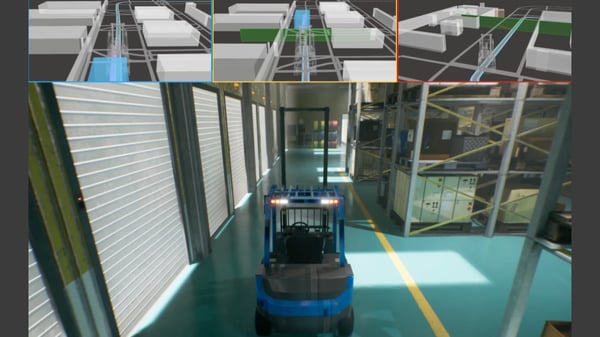
In this image, the AV sees its mapped route (represented by the blue path line), and recognizes fixed objects in the facility (represented as grey blocks).
For example, as an autonomous vehicle moves through a warehouse, it can extract features such as edges from a pillar, enabling the vehicle to recognize that the object is static and useful for the AV to determine where it is in the warehouse.
Conversely, when the vehicle detects features from a moving forklift, AI helps it understand that this object is dynamic and that it should be accounted for by the perception system. In turn, the AV will omit the data points identified as “forklift” from the localization system since a moving object could compromise localization accuracy.
-
How does AI contribute to a cheaper and faster deployment bring-up for customers?
With the help of AI, Cyngn can also utilize semantic segmentation and other ML-based automations to provide customers with a quicker and more cost-effective deployment process.
In large or complex environments, the mapping process can be nontrivial. However, AI-powered tools allow Cyngn to create high-resolution maps of your factory floor, warehouse, and other industrial settings, in just a few hours. These high-resolution maps provide Cyngn AVs with the centimeter-level precision that is pivotal in enabling self-driving solutions that efficiently navigate your site how you need them to, instead of constantly getting stuck and asking for help.
How does this work? Semantic segmentation enables us to segment drivable regions, such as corridors, in addition to exclusionary zones, so that vehicles can better navigate their environments. This significantly reduces the cost and time required to deploy AVs in industrial settings, making it easier and more efficient for companies to integrate these technologies into their workflows.
Moreover, these maps can be updated systematically by using the data that’s already being collected by the vehicles while they operate, allowing the vehicles to adapt to changes in the environment, such as the movement of objects or changes in layouts.